Train & improve high-quality, fast models
Fine-tune open-source models with your data to create task-specific, fast, cheap models that are 100% yours.
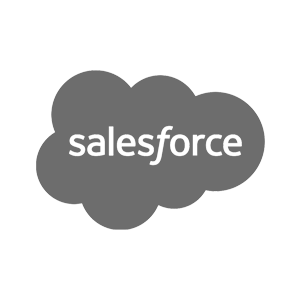
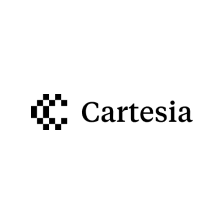
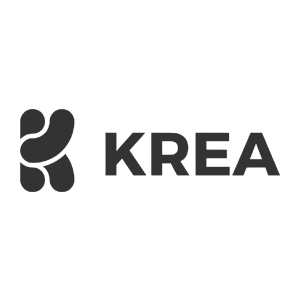
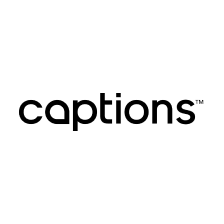
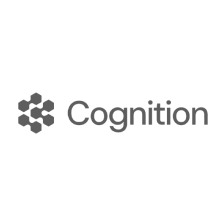
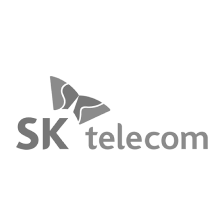
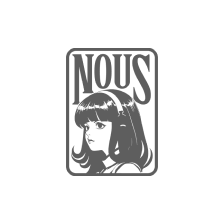
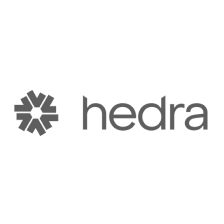
Custom models that are faster, more accurate, cheaper, and 100% yours
Together Fine-Tuning allows you to train open-source models with your data to create models that excel at specific tasks, match your tone of voice & more.
Task-specific models
Improve overall quality by fine-tuning a model to provide high-quality results customized for specific tasks and domains.
Smaller & faster at lower cost
Create smaller fine-tuned models that match the quality of large models with much faster performance and lower cost.
Deploy & download
Once your model is created, seamlessly deploy and run inference on Together Cloud or download the resulting checkpoint.
"After thoroughly evaluating multiple LLM infrastructure providers, we’re thrilled to be partnering with Together AI for fine-tuning. The new ability to resume from a checkpoint combined with LoRA serving has enabled our customers to deeply tune our foundation model, ShieldLlama, for their enterprise’s precise risk posture. The level of accuracy would never be possible with vanilla open source or prompt engineering."
- Alex Chung, Founder of Protege AI
Train models that adapt & evolve with your users
Fine-tune leading open-source models to capture your domain expertise and continuously adapt them as your app evolves.
Run LoRA or full fine-tuning jobs
Start full fine-tuning jobs to create new custom models trained on your data.
For a faster, memory-efficient approach, use LoRA fine-tuning, which produces small adapters you can use when running inference.
Meet user preferences with Direct Preference Optimization
Align models with human preferences using preferred vs. non-preferred responses to improve output quality.
By training directly on preferences, DPO teaches your model to distinguish good responses from bad ones, yielding more helpful, human-centric outputs.
Improve over time with Continued Fine-Tuning
Adapt an already fine-tuned LLM to new tasks, languages or data without losing the skills it has already learned.
Building on an existing model’s knowledge saves time and compute resources versus training from scratch.
Continued fine-tuning guards against catastrophic forgetting, preserving prior strengths while adding new skills.
Deploy seamlessly to Together Cloud
Get started
Read our quickstart guides to start fine-tuning models with your own data today.